Optimizing dataset diversity for a robust deep-learning model in rice blast disease identification to enhance crop health assessment across diverse conditions
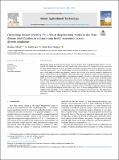
View/ Open
Date
2025-03Author
Alfred, Reuben
Leo, Judith
Kaijage, Shubi
Metadata
Show full item recordAbstract
Magnaporthe oryzae, the pathogen that causes rice blast disease, poses a significant global threat to rice pro
duction. This disease may lead to yield losses exceeding 30 % in susceptible rice varieties. There is an urgent need
for more effective detection solutions, as traditional methods—primarily based on visual inspection—are time-
consuming and prone to errors. Deep-learning models presented effective solutions for disease identification
due to their ability to analyze large datasets. However, the diversity of the training dataset is significant for
optimal performance and generalizability of the model. This study evaluated the impact of dataset diversity on
model performance and generalizability by developing two models, referred to in this study as the High-Diverse
Model and the Low-Diverse Model. The High-Diverse Model was trained on a diverse dataset comprising images
from different geographical regions, rice species, environmental conditions, plant growth stages, and disease
severity levels. In contrast, the Low-Diverse Model was trained on a less diverse dataset with significantly limited
variability. The results showed that the High-Diverse Model significantly outperformed the Low-Diverse Model,
achieving a training accuracy of 95.26 % and a validation accuracy of 94.43 %, indicating effective general
ization. The Low-Diverse Model achieved an accuracy of 98.37 % on the training data but only 35.38 % on the
validation data, indicating a severe overfitting issue associated with limited dataset diversity. This highlights the
importance of dataset diversity in developing effective and scalable deep-learning models for crop health
assessment.
URI
https://doi.org/10.1016/j.atech.2024.100726https://dspace.nm-aist.ac.tz/handle/20.500.12479/2998