Characterizing Water Users through Frequent Patterns and Association Rules by Using Apriori Algorithm: A Case of Pangani Basin Tanzania
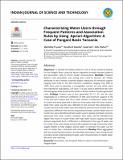
View/ Open
Date
2024-12-07Author
Lyuba, Matimbila
Nyambo, Devotha
Sam, Anael
Tilahun, Seifu
Metadata
Show full item recordAbstract
Objectives: To identify the hidden patterns in the K-means clustered dataset
for the Pangani Basin using the Apriori algorithm through frequent patterns
and association rules to enrich cluster characteristics. Methods: Frequent
patterns and association rule mining were used to discover the hidden
attributes in the K-means clustered dataset. Measures of minimum support
ranging from 0.5% to 5% and minimum confidence ranging from 50% to
100% were used to generate a manageable number of rules which were
then filtered for redundancy. Lift value >1.0 was used to determine the rule’s
interestingness while Arules and ArulesViz in R were used to visualize generated
rules. Findings: Clusters one to four generated 25, 31, 47, and 49 rules
respectively at a minimum confidence of 50% and minimum support of 2%
in the first two clusters and 1% in other clusters. Furthermore, water users
in cluster one were observed to abstract more water than the three clusters,
while their water use fee also reflected on the amount they abstracted. In
clusters two and three, water users identified the same amount of water source
capacity but differed in the amount requested and water use fee. Water users in
cluster four were identified with less water source capacity and fewer amounts
abstracted than other clusters. However, their water use fee identified was
higher than those in cluster three, with high water source capacity and high
amount requested. Such a difference is attributed to the type of water use
for cluster three users being domestically supplied through community water
supply entities to help villagers access water. In contrast, the water use for
users in cluster four is domestic and commercial. Novelty: When aggregated
with the clustering observations, the identified association rules mining results
provide a broad understanding of water users’ characteristics for better water
allocation and rationing.