Predicting customer subscription in bank telemarketing campaigns using ensemble learning models
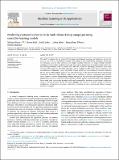
View/ Open
Date
2025-03Author
Peter, Michael
Mofi, Hawa
Likoko, Said
Sabas, Julius
Mbura, Ramadhani
Mduma, Neema
Metadata
Show full item recordAbstract
This study investigates the use of ensemble learning models bagging, boosting, and stacking to enhance the accuracy and reliability of predicting customer subscriptions in bank telemarketing campaigns. Recognizing the challenges posed by class imbalance and complex customer behaviors, we employ multiple ensemble techniques to build a robust predictive framework. Our analysis demonstrates that stacking models achieve the best overall performance, with an accuracy of 91.88% and an Receiver Operating Characteristic Area Under the Curve (ROC-AUC) score of 0.9491, indicating a strong capability to differentiate between subscribers and non-subscribers. Additionally, feature importance analysis reveals that contact duration, economic indicators like the Euro interbank offered (Euribor) rate, and customer age are the most influential factors in predicting subscription likelihood. These findings suggest that by focusing on customer engagement and economic trends, banks can improve telemarketing campaign effectiveness. We recommend the integration of advanced balancing techniques and real-time prediction systems to further enhance model performance and adaptability. Future work could explore deep learning models and interpretability techniques to gain deeper insights into customer behavior patterns. Overall, this study highlights the potential of ensemble models in predictive modeling for telemarketing, providing a data-driven foundation for more targeted and efficient customer acquisition strategies.
URI
https://doi.org/10.1016/j.mlwa.2025.100618https://dspace.nm-aist.ac.tz/handle/20.500.12479/2852