Frequentist and Bayesian Approaches in Modeling and Prediction of Extreme Rainfall Series: A Case Study from Southern Highlands Region of Tanzania
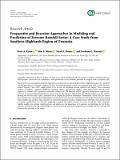
View/ Open
Date
2024-01-30Author
Kyojo, Erick
Mirau, Silas
Osima, Sarah
Masanja, Verdiana
Metadata
Show full item recordAbstract
This study focuses on modeling and predicting extreme rainfall based on data from the Southern Highlands region, the critical for
rain-fed agriculture in Tanzania. Analyzing 31 years of annual maximum rainfall data spanning from 1990 to 2020, the Gen-
eralized Extreme Value (GEV) model proved to be the best for modeling extreme rainfall in all stations. Tree estimation
methods–L-moments, maximum likelihood estimation (MLE), and Bayesian Markov chain Monte Carlo (MCMC)–were
employed to estimate GEV parameters and future return levels. Te Bayesian MCMC approach demonstrated superior per-
formance by incorporating noninformative priors to ensure that the prior information had minimal infuence on the analysis,
allowing the observed data to play a dominant role in shaping the posterior distribution. Furthermore, return levels for various
future periods were estimated, providing guidance for food protection measures and infrastructure design. Trend analysis using p
value, Kendall’s tau, and Sen’s slope indicated no statistically signifcant trends in rainfall patterns, although a weak positive trend
in extreme rainfall events was observed, suggesting a gradual and modest increase over time. Overall, the study contributes
valuable insights into extreme rainfall patterns and underscores the importance of L-moments in identifying the best ft dis-
tribution and Bayesian MCMC methodology for accurate parameter estimation and prediction, enabling effective measures and
infrastructure planning in the region.