A Survey of Machine Learning Modelling for Agricultural Soil Properties Analysis and Fertility Status Predictions
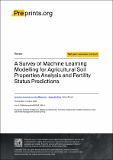
View/ Open
Date
2023-08-21Author
Malamsha, Augustine
Dida, Mussa
Moebs, Sabine
Metadata
Show full item recordAbstract
The problem of low soil fertility and limited research in agricultural data driven tools, may lead to
low crop productivity which makes it imperative to research in applications of high throughput computational
algorithms such as of machine learning (ML) for effective soil analysis and fertility status prediction in order
to assist in optimal soil fertility management decision-making activities. However, difficulties in the choice of
the key soil properties parameters for use in reliable soil nutrients analysis and fertility prediction. Also,
individual ML algorithms setbacks and modelling expert implementation procedures subjectivity, may lead to
exploitation of worst fertility level targets and soil fertility status targets classification models performance
reported variations. This paper surveys state-of-affair in ML for agricultural soil nutrients analysis and fertility
status prediction. Prominent soil properties and widely used classical modelling algorithms and procedures
are identified. Empirically exploited fertility status target classes are scrutinized, and reported soil fertility
prediction model performances are depicted. The three pass method, with mixed method of qualitative content
analysis and qualitative simple descriptive statistics were used in this survey. Observably, the frequently used
soil nutrients and chemical properties were organic carbon, phosphorus, potassium, and potential Hydrogen,
followed by iron, manganese, copper and zinc. Predominant algorithms included Random Forest, and Naïve
Bayes, followed by Support Vector Machine. Model performances varied, with highest accuracy 98.93% and
98.15% achieved by ensemble methods, and the least being 60%. Interdisciplinary ML related researchers may
consider using ensemble methods to develop high performance soil fertility status prediction models.
URI
https://doi: 10.20944/preprints202308.1395.v1https://dspace.nm-aist.ac.tz/handle/20.500.12479/1950